Can you briefly explain what your project is all about? What’s unique about it?
The LANGSWITCH project aims to develop socially acceptable ASR (Automatic Speech Recognition) systems suitable for use in environments and agents such as those of the SERMAS project. These ASR systems must work in real-time, perform well in noisy environments, distinguish between different speakers, respect the privacy of the user, and work in a variety of languages with very different characteristics (large, less-resourced…).
What’s the biggest milestone with your project your startup(s) have achieved so far, and what has surprised you most on this journey?
So far we have worked with English, Spanish and Basque. For English, we have outperformed a high-quality, state-of-the-art system such as Whisper in very demanding noise conditions. For Spanish and Basque, we have improved on Whisper’s results in general and also in noisy conditions.
How did you measure success?
To measure the performance of our system, we use the standard WER (Word Error Rate) indicator, which is the percentage of words that are not correctly transcribed. We measure it in different noise conditions: no noise at all and different SNRs (Signal Noise Ratios), ranging from 10 dB (quite high ambient noise) to 0 dB (noise at the same volume as the speech). For English, we aimed to obtain a WER of around 5% in noisy conditions. We obtained 5.13, 5.65 and 7.21% in the 10, 8 and 5 dB conditions respectively by fine-tuning the Whisper large model and improving its results by one to two points. The results obtained by the Whisper small model and our system developed by fine-tuning it are worse, but the improvement over the base Whisper small model is greater, from two to six points. For the other languages, the results we obtain are similar, but the improvements over the base Whisper models are greater because the base Whisper models do not perform as well for these languages.
What are your goals over the next three and six, months?
Over the next six months, we plan to develop ASR systems that perform similarly in noisy environments in two more languages, French and Italian, as well as a speaker identification system that respects user privacy.
How has SERMAS helped you during the past few months?
The mentoring provided by SERMAS has been very helpful, as they have provided us with detailed use cases of ASR systems in real-world scenarios, thus pointing out the direction and specifics of our developments.
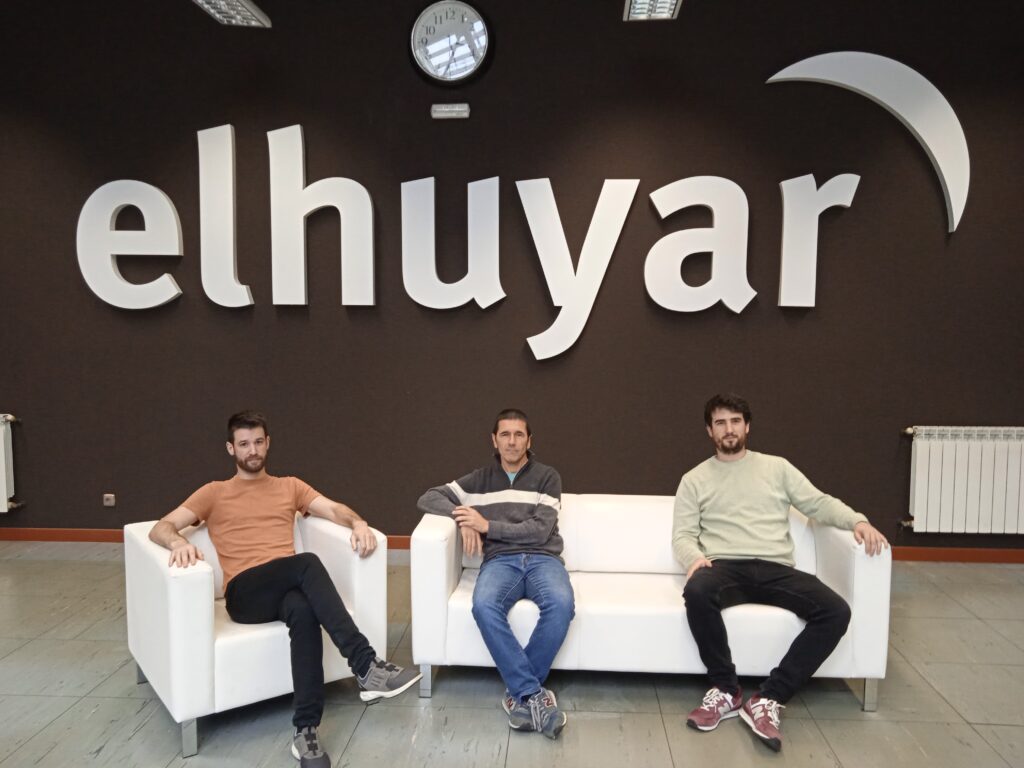
Company: ORAI NLP Teknologiak (Elhuyar Fundazioa)